How was this content?
How climate tech startups use generative AI to address the climate crisis
Generative artificial intelligence (AI) can seem like magic or like a clever collaborator, generating original text, images, videos, and music. It has grabbed the world’s attention with incredible chat capabilities and captivating image creation. However, it can be more than a creative collaborator or a chat bot. At AWS, we are seeing generative AI transform how humans and business use technology to solve some of the world’s most challenging problems.
There are few problems more urgent than the climate crisis. The world is in a race to get to net zero carbon emissions by 2050 to curb global warming to 2 degrees Celsius before the impact of climate change becomes irreversible. Speed is critical to addressing the climate crisis— generative AI is still an emerging field, but it is already becoming an important tool to accelerate the build and deployment of climate solutions.
We are excited to introduce you to a few Climate Tech startups that are at the forefront of the race to stop the climate crisis. They’re using generative AI to fight climate change by reducing greenhouse gas emissions and enabling the world to transition to a zero-carbon economy.

BrainBox AI: Accelerating building decarbonization through generative AI
According to the International Energy Agency (IEA), buildings account for 30 percent of global energy consumption and 26 percent of global energy-related emissions. Reducing buildings’ energy use is critical for getting to net zero global emissions.
BrainBox AI has developed autonomous AI to decarbonize and optimize commercial buildings. It also saves customers money on their energy bills. The cloud-based optimization solution built on AWS connects to buildings’ existing HVAC (heating, ventilation, and air conditioning) systems and autonomously sends real time optimized control commands to minimize emissions and energy consumption without any human intervention.
For example, BrainBox AI has helped building owners reduce HVAC energy costs by up to 25 percent and reduce HVAC-related greenhouse gas emissions by up to 40 percent by predicting the temperature in a retail store based on historical data and external datasets, like weather and energy tariff structures.
As BrainBox AI adds new buildings to their system, they utilize generative AI to reduce onboarding time for each new building. In the past, whenever a new piece of equipment was identified in a building, such as a pump or an air handling unit, engineers had to go through the complex technical manuals from the manufacturer, find details like the pump’s power rating or the pressure it generates, and finally convert that information into a machine-readable format.
Using Amazon Bedrock, BrainBox AI extracts data and generates configuration files automatically. These files are then completed and revised by engineers. This process is known as power tagging. With Amazon Bedrock, BrainBox AI estimates they reduced the time it takes to power tag by over 90 percent. As a result, BrainBox AI is able to onboard more customers, more quickly, so it can have a bigger, faster impact on the climate crisis.
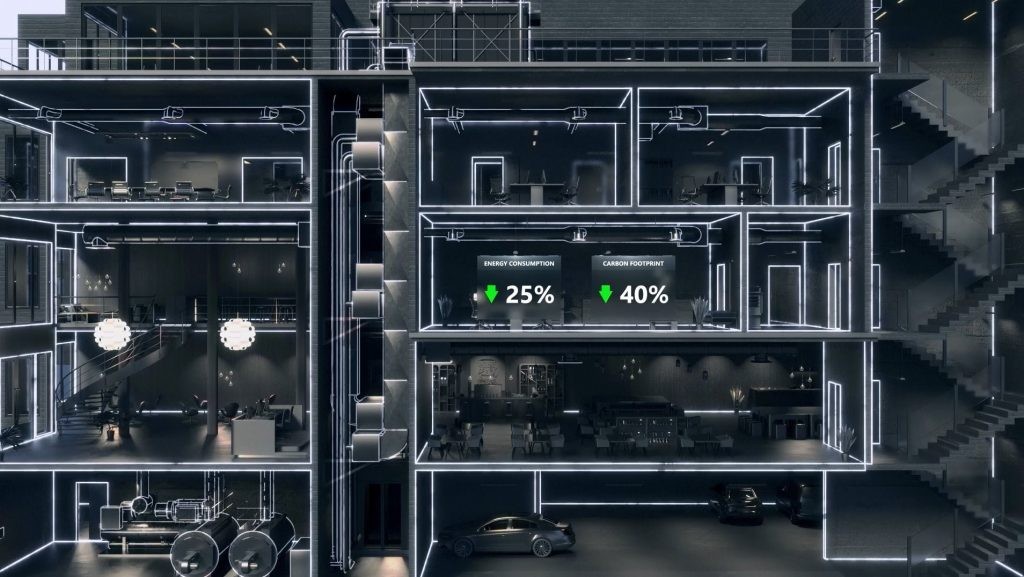

Pendulum: Decarbonizing the supply chain with generative AI
Pendulum harnesses the power of AI to address one of the world’s most pressing problems: how organizations can create more from less. The company’s technology offers sustainable solutions for complex problems in sectors such as commercial supply chain, global health, and national security.
Optimizing supply chains is crucial to reducing carbon emissions. Accenture estimates that supply chains generate 60 percent of all carbon emissions globally. According to the U.S. Environmental Protection Agency, supply chains can account for more than 90 percent of a company’s greenhouse gas emissions. When you look at how supply chains work (or don’t work) today, there are some startling details about the extent of wasted resources and capital. For instance, every year, an estimated $562 billion is lost in overstock, with 17 percent of food products and 8 percent of retail and consumer packaged goods products being discarded.
Pendulum’s AI-powered solutions enable organizations to intelligently manage their operations and reduce product waste, revenue loss, and excess greenhouse gas emissions. Built on AWS, Pendulum’s software can predict demand, plan supply, and geolocate shipments. It allows companies to more accurately purchase the resources they need while producing exactly the amount of goods their customers demand.
Accessing enterprise data is critical for Pendulum’s platform. However, data is often retained in siloed systems and unstructured documents such as PDF and plain text files. Pendulum’s software is designed to leverage the data sources most relevant to operational decision-making. They are deploying generative AI to rapidly unlock important information contained in long and complicated documents so they can accelerate time to value for their customers.
One example of where this is being effectively deployed is in precision agriculture. The Pendulum team uses a human-in-the-loop approach to instruction-tune a large language model (LLM) on AWS Trainium using Amazon SageMaker. This generates machine-readable data from unstructured files that their customers’ farming machinery can use to determine how much pesticide, water, and other products to use. As a result, the customer is less likely to overuse or over-order resources, can save money, and reduce their carbon footprint and environmental impact. Furthermore, it allows customers to adhere to plant needs, local regulations, and other important criteria.
Pendulum estimates this solution has reduced the time required to decode these documents by 83 percent, and they now only need to review the data for quality assurance. This in turn reduces costs and accelerates the deployment of their software at scale.
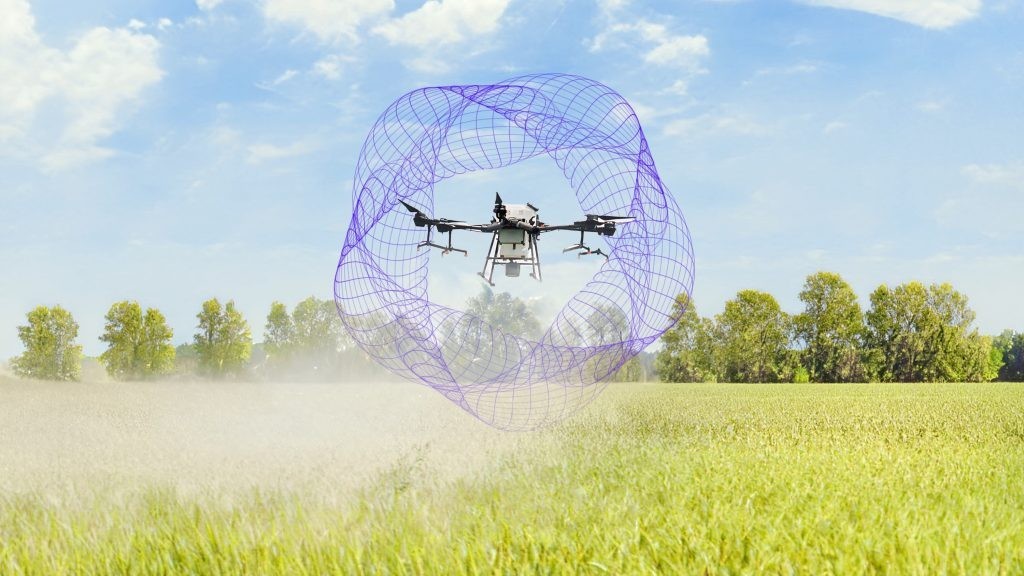
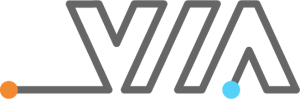
VIA: Making it easier for building managers to understand energy efficiency with generative AI
To enable emissions reduction, institutions and businesses need to track energy data at the local and individual level. For instance, in order to reduce the carbon emissions associated with its fleet of electric vehicles (EVs), it’s important for a company to understand if EVs, in a certain region, at a certain time, are charged using electricity powered by renewables or fossil fuels. For energy efficient buildings, in-depth individualized data across an organization’s entire real estate portfolio is essential. If everyone provided all data transparently, this wouldn’t be a problem. However, individual-level data is often not accessible because of privacy issues or security concerns. Many individuals are reluctant to provide the time, date, and location of their vehicle charging/discharging/energy data. This makes energy management and greenhouse gas reduction challenging.
Via Science, Inc. (VIA) enables organizations to reduce their carbon footprint as a collective, while keeping individual data private and secure. The company provides sustainability data using zero-knowledge proofs tested and verified by the U.S. Department of Energy. This enables organizations and businesses to track data and meet sustainability goals even when it’s not possible to share detailed information due to regulatory or privacy barriers.
VIA initially developed a solution for the U.S. Air Force, which has strict data privacy requirements that often prohibits building management and energy management teams from accessing critical data they need. VIA’s decentralized software solution enables airmen and permitted contractors to use generative AI models without sharing data: no private data is used to train the model or sent to the model in the prompt. Instead, when a user enters a prompt like “show me all buildings on Air Force Base XYZ with HVAC system condition less than 60,”, the LLM responds with “I understand what you want to achieve, and, because I don’t have access to the data, I will generate a SQL query that you can run to get the data from your local database. I will also send you the frontend code you can run to display the data.” These two pieces of code are then sent back to the user where the tool, SLAM AI, automatically runs and visualizes the data locally.
To further save energy and reduce compute costs, VIA uses compact open-source LLMs that run on CPUs. They continually assess new models due to the rapid evolution of LLM performance. Leveraging Amazon Elastic Kubernetes Service (EKS), they can seamlessly hot swap models, integrating more efficient ones as they become available.
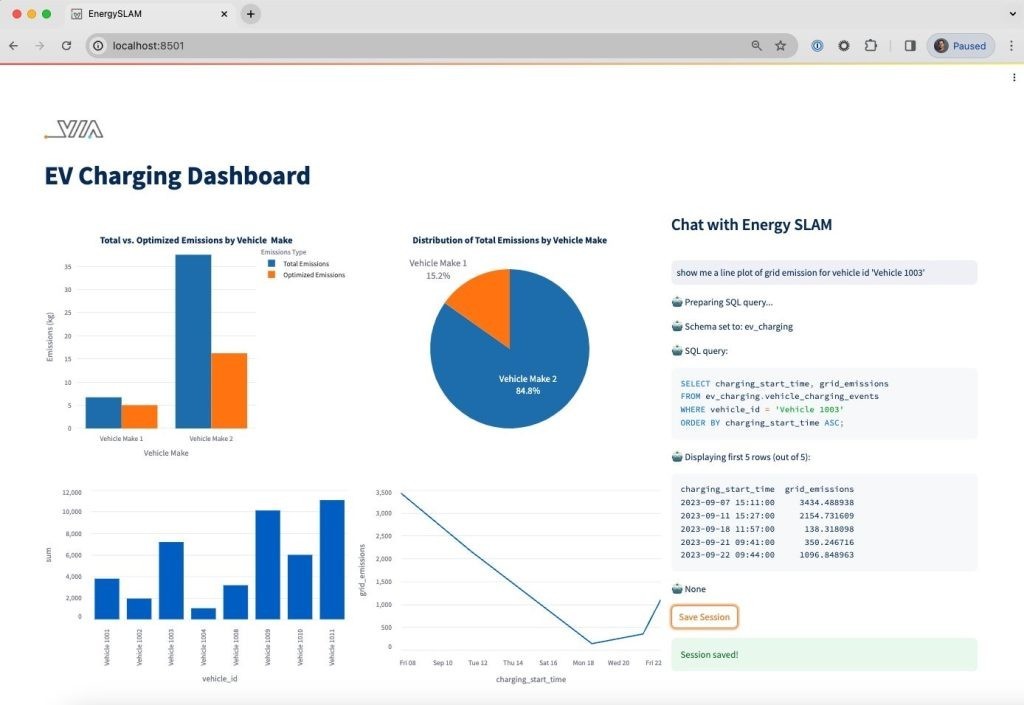
What’s next for generative AI and climate tech
BrainBox AI, Pendulum, and VIA are using generative AI on AWS in exciting ways to address the climate crisis. They make use of generative AI’s ability to extract key elements from unstructured data and generate new content. This enables these companies to serve their customers more quickly, serve more customers, and reduce greenhouse gas emissions. It also reduces costs for these companies and for their customers.
We expect that Climate Tech startups will find additional new ways to use generative AI on AWS to address the climate crisis. Here are a few examples of what we are seeing in other industries that we think could apply to Climate Tech.
Data augmentation using generative AI to generate synthetic data for predictive model training
Generative Al can create synthetic data, which is a class of data that is generated rather than obtained from direct observations of the real world. This could be useful for subsurface modeling for geothermal or carbon sequestration where subsurface rock formation data is hard to come by. Startups in low-carbon transportation could also use generative AI to create scenarios to test new vehicles. It could also be useful in Climate Tech hard-tech manufacturing. Synthetic image data creation can be used for creating images of equipment (e.g., compressors, turbines) with rust or cracks. These images can be used for training vision-based machine learning (ML) models for predictive maintenance, which can play a key role in reducing costs and minimizing operational downtime.
Improve Climate Tech manufacturing efficiency using generative AI
By using models trained on historical data, including machine usage and maintenance logs, generative AI can identify patterns and links between various factors, such as temperature, vibration, and operating hours. This can enable the system to foresee the likelihood of equipment failure and proactively communicate those patterns to the right stakeholders such as quality engineers, maintenance engineers, and operators. By proactively communicating the need for maintenance, downtime will be reduced, minimizing disruptions to manufacturing.
Design and synthesize new protein sequences for sustainable agriculture and food production with generative AI
Generative AI can predict protein folded structures that enable them to carry out particular functions in the cell. This will allow researchers to generate functional proteins and different molecules in a guided fashion. Additionally, generative AI allows scientists to accurately define the structure of known protein sequences to identify molecular/biological targets.
There are likely many more ways that Climate Tech startups can use generative AI to address global warming. We hope this blog post sparks ideas and inspires Climate Tech founders to use generative AI in new and exciting ways.
Generative AI workloads can consume large amounts of energy and cloud resources, and as with all workloads, it is essential to consider their environmental impact. It’s our collective responsibility to make sustainable use of this technology. Amazon is committed to reaching net-zero carbon by 2040. As part of this commitment, Amazon is on a path to powering its operations with 100 percent renewable energy by 2025, including AWS data centers. This has led to Amazon being the world’s largest corporate buyer of renewable energy for the last four years. AWS provides guidance to help companies optimize their generative AI workloads for environmental sustainability. It is also critical that these companies measure the impact of their use of generative AI and its contribution to the overall sustainability goals of the organization.
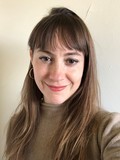
Lisbeth Kaufman
Lisbeth Kaufman is the Head of Climate Tech BD, Startups and Venture Capital at AWS. Her mission is to help the best Climate Tech startups succeed and reverse the global climate crisis. Her team has technical resources, go to market support and connections to help climate tech startups overcome obstacles and scale. Lisbeth was Founder and CEO of KitSplit.com, a sharing economy company called “the Airbnb of Cameras” by Forbes. Before she was a founder, Lisbeth worked on climate policy as an energy/environment/agriculture policy advisor in the U.S. Senate. There she built a first-of-its-kind energy efficiency retrofit program and wrote a clean energy bill for farmers that got passed into law. Lisbeth has a BA from Yale and an MBA from NYU Stern where she was a Dean's Scholar. As a mentor at Techstars and an EIR a Entrepreneurs Roundtable Accelerator Lisbeth helps climate tech founders with product, growth, fundraising, as well as making strategic connections to teams at AWS and Amazon.
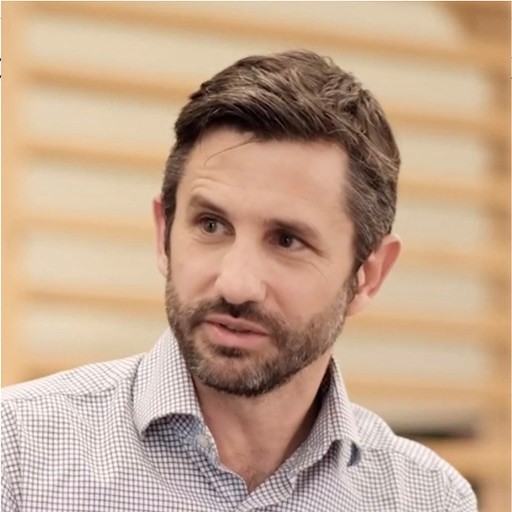
Benoit de Chateauvieux
Benoit de Chateauvieux is a Startup Solutions Architect at AWS, based in Montreal, Canada. As a former CTO, he enjoys helping startups build great and sustainable products using the cloud. Outside of work, you’ll find Benoit in canoe-camping expeditions, paddling across Canadian rivers.
How was this content?