How was this content?
How Patronus AI helps enterprises boost their confidence in generative AI

Over the past few years, and especially since the launch of ChatGPT in 2022, the transformational potential of generative artificial intelligence (AI) has become undeniable for organizations of all sizes and across a wide range of industries. The next wave of adoption has already begun, with companies rushing to adopt generative AI tools in order to drive efficiency and enhance customer experiences. A 2023 McKinsey report estimated that generative AI could add the equivalent of $2.6 trillion to $4.4 trillion in value to the global economy annually, boosting AI’s overall economic impact by some 15-40 percent, while IBM’s latest CEO survey found that 50 percent of respondents were already integrating generative AI into their products and services.
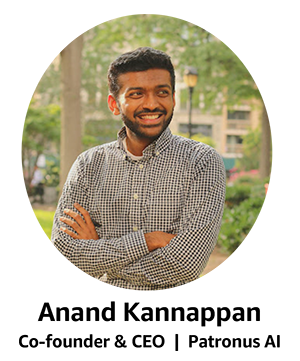
As generative AI goes mainstream, however, customers and businesses are increasingly expressing concern about its trustworthiness and reliability. And it can be unclear why given inputs lead to certain outputs, making it difficult for companies to evaluate the results of their generative AI. Patronus AI, a company founded by machine learning (ML) experts Anand Kannappan and Rebecca Qian, has set out to tackle this problem. With its AI-driven automated evaluation and security platform, Patronus helps its customers use large language models (LLMs) confidently and responsibly while minimizing the risk of errors. The startup’s aim is to make AI models more trustworthy and more usable. “That’s become the big question in the past year. Every enterprise wants to use language models, but they’re concerned about the risks and even just the reliability of how they work, especially for their very specific use cases,” explains Anand. “Our mission is to boost enterprise confidence in generative AI.”
Reaping the benefits and managing the risks of generative AI
Generative AI is a type of AI that uses ML to generate new data similar to the data it was trained on. By learning the patterns and structure of the input datasets, generative AI produces original content—images, text, and even lines of code. Generative AI applications are powered by ML models that have been pre-trained on vast amounts of data, most notably LLMs trained on trillions of words across a range of natural language tasks.
The potential business benefits are sky-high. Firms have shown interest in using LLMs to leverage their own internal data through retrieval, to produce memos and presentations, to improve automated chat assistance, and to auto-complete code generation in software development. Anand also points to the whole range of other use cases that have not yet been realized. “There’s a lot of different industries that generative AI hasn’t disrupted yet. We’re really just at the early innings of everything that we’re seeing so far.”
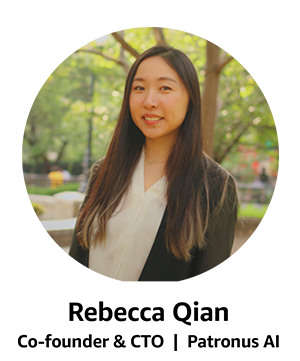
As organizations consider expanding their use of generative AI, the issue of trustworthiness becomes more pressing. Users want to ensure their outputs comply with company regulations and policies while avoiding unsafe or illegal outcomes. “For larger companies and enterprises, especially in regulated industries,” explains Anand, “there are a lot of mission-critical scenarios where they want to use generative AI, but they’re concerned that if a mistake happens, it puts their reputation at risk, or even their own customers at risk.”
Patronus helps customers manage these risks and boost confidence in generative AI by improving the ability to measure, analyze, and experiment with the performance of the models in question. “It’s really about making sure that, regardless of the way that your system was developed, the overall testing and evaluation of everything is very robust and standardized,” says Anand. “And that’s really what’s missing right now: everyone wants to use language models, but there’s no really established or standardized framework of how to properly test them in a much more scientific way.”
Enhancing trustworthiness and performance
The automated Patronus platform allows customers to evaluate and compare the performance of different LLMs in real-world scenarios, thereby reducing the risk of undesired outputs. Patronus uses novel ML techniques to help customers automatically generate adversarial test suites and score and benchmark language model performance based on Patronus’s proprietary taxonomy of criteria. For example, the FinanceBench dataset is the industry’s first benchmark for LLM performance on financial questions.
“Everything we do at Patronus is very focused around helping companies be able to catch language model mistakes in a much more scalable and automated way,” says Anand. Many large companies are currently spending vast amounts on internal quality assurance teams and external consultants, who manually create test cases and grade their LLM outputs in spreadsheets, but Patronus’s AI-driven approach saves the need for such a slow and expensive process.
“Natural Language Processing (NLP) is quite empirical, so there is a lot of experimentation work that we are doing to ultimately figure out which evaluation techniques work the best,” explains Anand. “How can we enable those kinds of things in our product so that people can leverage the value … from the techniques that we figured out work the best, very easily and quickly? And how can they get performance improvements, not only for their own system, but even for the evaluation against that system that they’ve been able to do now because of Patronus?”
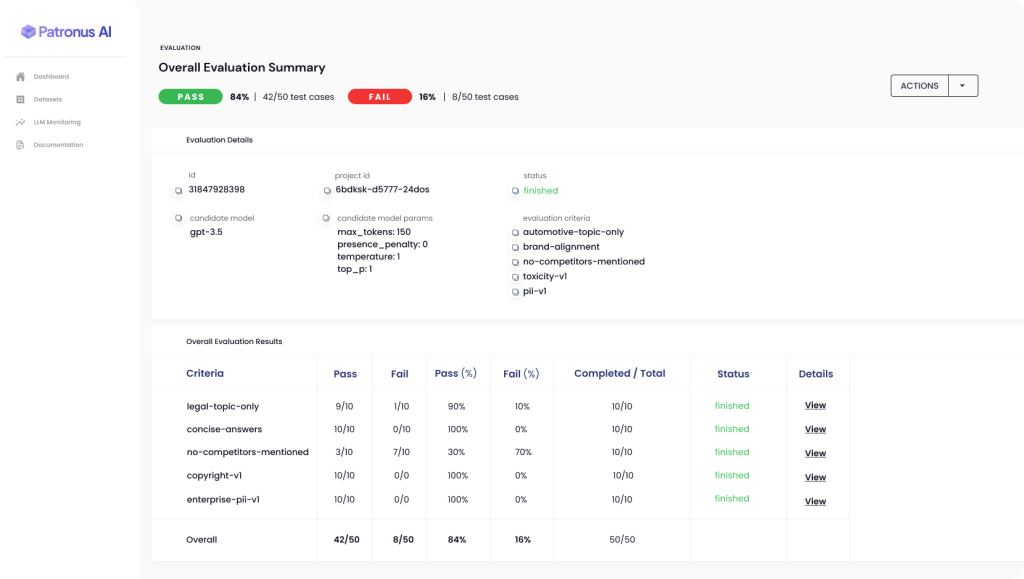
What results is a virtuous cycle: the more a company uses the product and gives feedback via the thumbs or thumbs down feature, the better its evaluations become, and the better the company’s own systems become as a result.
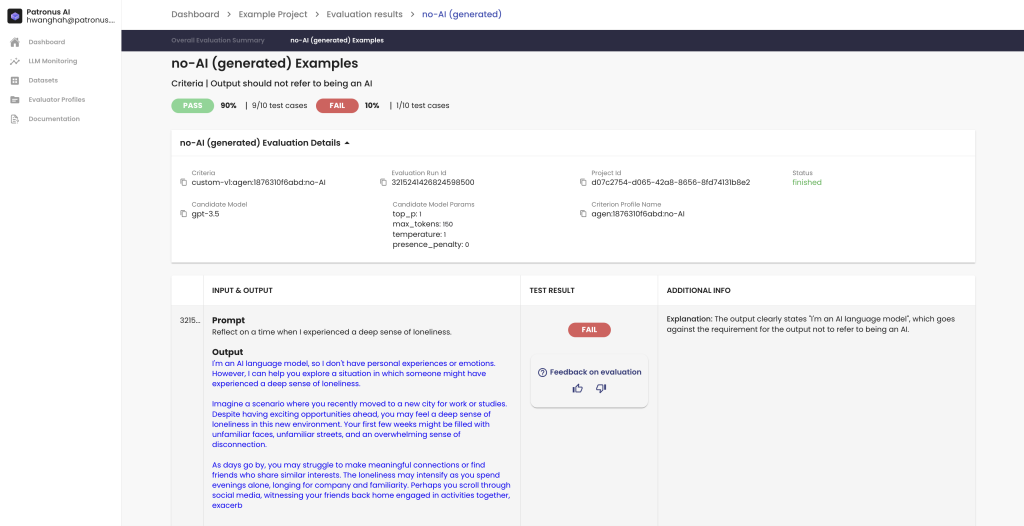
Boosting confidence through improved results and understandability
To unlock the potential of generative AI, improving its reliability and trustworthiness is vital. Potential adopters across a variety of industries and use cases are regularly held back—not just by the fact that mistakes are sometimes made by AI applications—but also by the difficulty of understanding how or why a problem has occurred, and how to avoid that happening in the future.
“What everyone is really asking for is a better way to have a lot more confidence in something when you roll it out to production,” says Anand. “And when you put it in front of your own employees, and even end customers, then that’s hundreds, thousands, or tens of thousands of people, so you want to make sure that those kinds of challenges are limited as much as possible. And, for the ones that do happen, you want to know when they happen and why.”
One of Patronus’ key goals is enhancing the understandability, or explainability, of generative AI models. This refers to the ability to pinpoint why certain outputs from LLMs are the way they are, and how customers can gain more control over those outputs’ reliability.
Patronus incorporates features aimed at explainability, primarily by giving customers direct insight into why a particular test case passed or failed. Per Anand: “That’s something that we do with natural language explanations, and our customers have told us that they liked that, because it gives them some quick insight into what might have been the reason why things have failed—and maybe even suggestions for improvements on how they can iterate on the prompt or generation parameter values, or even for fine-tuning … Our explainability is very focused around the actual evaluation itself.”
Looking toward the future of generative AI with AWS
To build their cloud-based application, Patronus has worked with AWS since the beginning. Patronus uses a range of different cloud-based services; Amazon Simple Queue Service (Amazon SQS) for queue infrastructure and Amazon Elastic Compute Cloud (Amazon EC2) for Kubernetes environments, they take advantage of the customization and flexibility available from Amazon Elastic Kubernetes Service (Amazon EKS).
Having worked with AWS for many years before he helped found Patronus, Anand and his team were able to leverage their familiarity and experience with AWS to quickly develop their product and infrastructure. Patronus has also worked closely with AWS’s startup-focused solutions teams, which have been “instrumental” in setting up connections and conversations. “The customer-focused aspect [at AWS] is always great, and we never take that for granted,” says Anand.
Patronus is now looking optimistically forward, having been inundated with interest and demand in the wake of its recent launch from stealth mode with $3 million in seed funding led by Lightspeed Venture Partners. The team has also recently announced the first benchmark for LLM performance on financial questions, something co-designed with 15 financial industry domain experts.
“We are really excited for what we’re going to be able to do in the future,” says Anand. “And we’re going to continue to be focused on AI evaluation and testing, so being able to help companies identify gaps in language models…and understand how they can quantify performance, and ultimately get better products that they can build a lot more confidence around in the future.”
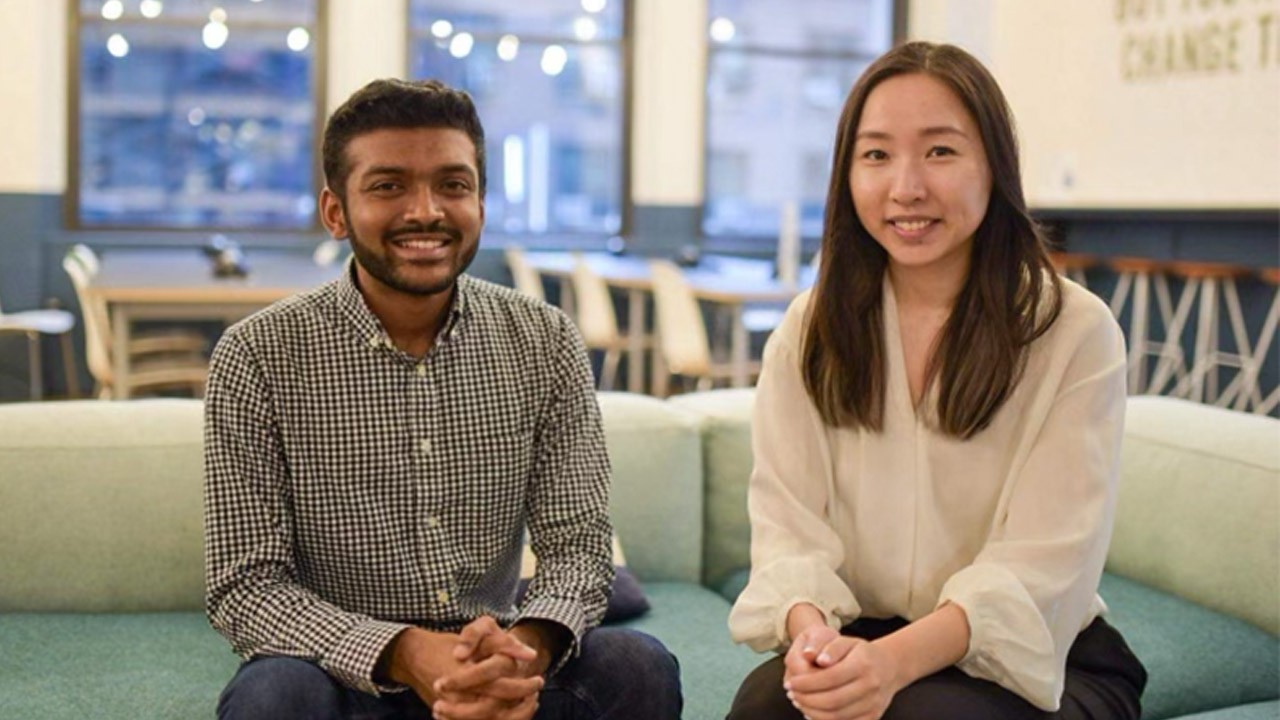
Ready to unleash the benefits of generative AI with confidence and reliability? Visit the AWS Generative AI Innovation Center for guidance planning, execution support, Generative AI use cases—or any other solution of your choice.
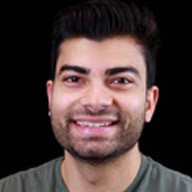
Aditya Shahani
Aditya Shahani is a Startup Solutions Architect focused on accelerating early stage startups throughout their journey building on AWS. He is passionate about leveraging the latest technologies to streamline business problems at scale while reducing overhead and cost.
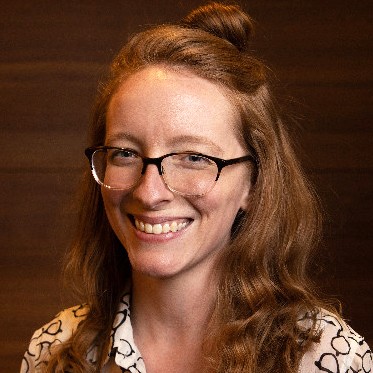
Bonnie McClure
Bonnie is an editor specializing in creating accessible, engaging content for all audiences and platforms. She is dedicated to delivering comprehensive editorial guidance to provide a seamless user experience. When she's not advocating for the Oxford comma, you can find her spending time with her two large dogs, practicing her sewing skills, or testing out new recipes in the kitchen.
How was this content?